13 Sep 2018 Opportunities and Limits of using Artificial Intelligence within Asset Management
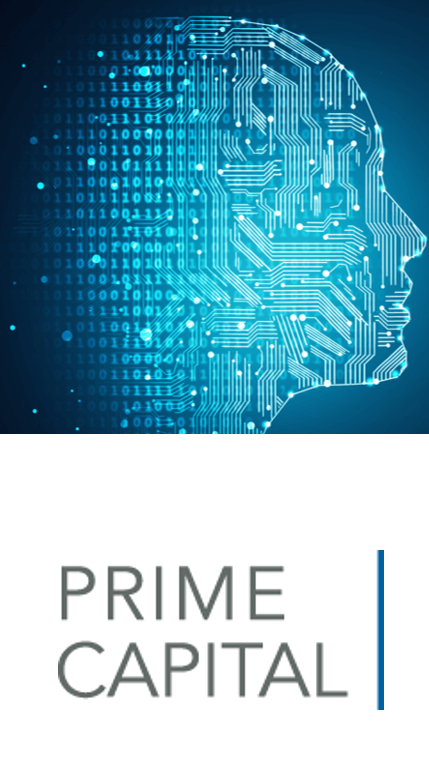
Vincent Weber is Managing Director of Absolute return at prime Capital and heads the research department. He is also lead portfolio manager of the Gateway Target Beta UCITS fund which is a quantitative investment strategy that mixes risk parity with CTA and Alternative Risk premia approaches. Vincent has a particular expertise in developing Prime capital’s systematic asset allocation and risk models.
Since the development of so-called robo-advisors, the concept of artificial intelligence (AI) has gained more and more attention. Often, AI is associated with terms such as machine learning (ML), deep learning, and big data, making the topic even more complex and difficult to grasp. Artificial intelligence can lead to new investment ideas and more robust portfolio allocations regardless of the implemented investment strategy. Indeed, this technology can benefit the entire spectrum of an asset management company’s value chain.
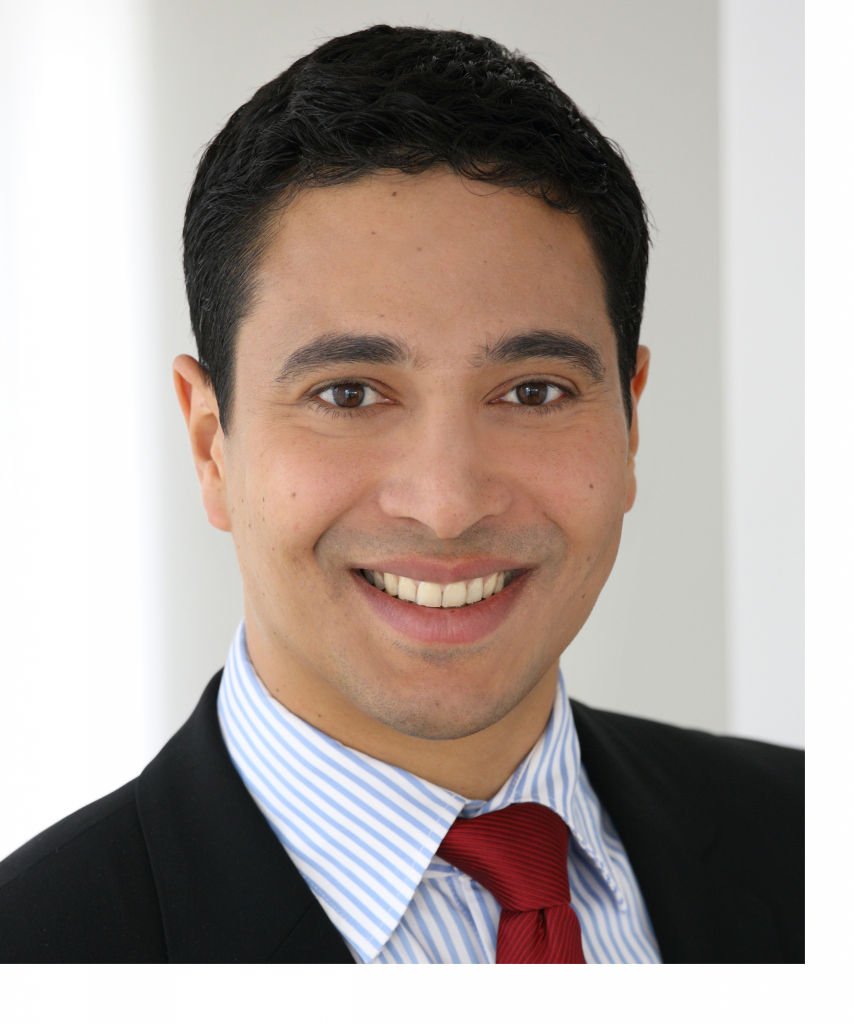
The idea of AI is certainly not new and the concept has been coined long before the beginning of the 21st century. Already in 1956, pioneering researchers at a Dartmouth college conference used the term to describe machines which possess the same characteristics as the human brain. Even back then the range of possibilities offered by “General AI” was fascinating, yet technological limitations made it impossible to materialize the concept. The optimal point still has not been reached, but the always evolving computer power has now mastered “narrow AI” which can be defined as a technology that is able to perform single specific tasks at least equally or sometimes even better than humans are. These tasks include face recognition, driving a car or classifying different images.
The inherent “intelligence” of these systems is thereby rooted in the methods of machine learning (ML). Contrary to widespread beliefs, ML is not equivalent to AI, but merely represents a subclass of it, such as calculus is a subclass of the overall field of mathematics.
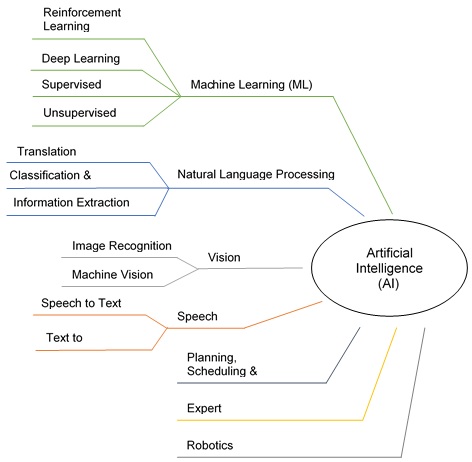
The essence of ML lies in the utilization of algorithms to analyse data, derive learnings from it and then predict outcomes. It combines statistical and computer science methods which can be subdivided into the following categories: supervised, unsupervised, reinforcement and deep learning.
In a “supervised learning” problem each input variable is assigned an output variable, it uses existing relationships among the observable data to establish a relation between input and output data. For example, a bank could use its customer’s income, overall wealth and family status to assess creditworthiness. Existing customer information is then calibrated and applied to the new situation.
On the contrary, “unsupervised learning” algorithms only use input data, there is no associated output data. Based on the existent output data, the algorithm attempts to uncover a relation between the observation points. Cluster analysis, which groups data with similar characteristics, is often implemented in this area. For example, stocks can be grouped using an algorithm according to their risk characteristics thus replacing the classical sector classification.
Combining methods used by both supervised and unsupervised learning, we enter the field of “reinforcement learning”. The algorithm thereby first tries to detect patterns on its own and then uses a feedback mechanism to make an informed decision. Such algorithms are at the basis of adaptive trading systems which are able to independently execute trades that can then be evaluated for gains or losses.
Finally, “deep learning” algorithms are based on the idea of neural networks. It is probably the area of AI which comes closest to replicating the functioning of the human nervous system. A neural network consists of different layers of neurons which are connected to other neurons that process input data through a non-linear function. Neural networks are nothing new. In fact, the topic has been discussed since the early stages of the AI revolution. However, all methods described above are very data consuming and require a tremendous amount of computing power, which so far have rendered their practical implementation rather inadequate. Still, with the increased availability of data and accessible computational power, these models have regained momentum in recent years.
With the increasing popularity of artificial intelligence, asset managers have included these techniques in their value chain to improve investment processes. For instance, an unsupervised learning algorithm, such as principal component analysis, could be used to derive a limited number of forecast indicators from a broad universe of financial market data. These new, algorithmically generated indicators could in turn be used in a second step by a “supervised learning” algorithm, such as linear regression, to forecast asset returns and generate a corresponding buy or sell signal.
However, most of the methods used today in asset management are based on “supervised learning”. In this respect, the classical models already developed in statistics are often sufficient to capture the majority of the trading signals generated by the underlying data. Since financial prices follow an almost random walk, the level of information on the basis of which new investment ideas can be developed is limited. Complex models that need a lot of information for accurate calibration are more likely to distort rather than identify actual new trends. Therefore we still have to wait until enough data is available for neural networks to be able to provide reliable forecasts. Although AI has gained importance in recent years, the investment process still requires expert knowledge to determine input variables and monitor models.